What's the Best Way to Adapt AI Methods for 3D Shapes?
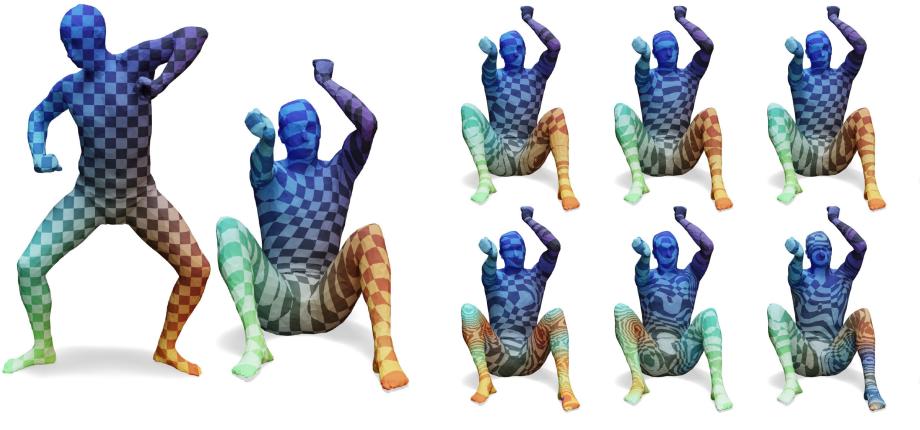
Whether it is medical MRI scans, three-dimensional scans of archaeological objects, computer-designed industrial parts or modelled environments for video games, 3D shapes are used in a wide range of fields. There is therefore a need to analyse, reconstruct, process or classify three-dimensional objects. Although tools to tackle these problems already exist, their design, and more fundamentally the analysis of this geometric data, remains a very active research topic. This is Maks Ovsjanikov's core speciality, within the GeomeriX team, set up in partnership with Inria at the École Polytechnique Computer Science Laboratory (LIX*). In particular, after receiving a Starting Grant from the European Research Council (ERC) in 2017 for the EXPROTEA project, Maks Ovsjanikov is now leading the VEGA project thanks to a Consolidator Grant.
Among the fundamental challenges in this field of research, a key task is to compare geometric shapes with each other, especially when they are not rigid objects. Can we tell whether two shapes are related in some way, whether they have parts that are similar or even identical? The researcher has, for instance, worked with palaeoanthropologists on comparisons of prehistoric human jaws to help reconstruct phylogenetic trees.
Transfert learning
Another crucial issue is to adapt artificial intelligence (AI) methods of machine learning to 3D geometric data. These methods have been very successful on 2D images, whether it is to recognise an object in a photograph, for example, or to generate new images. In the context of 3D data, generative models could allow the creation of innovative industrial objects by optimizing their geometric structure Maks Ovsjanikov is collaborating with several industrial companies in this direction.
In the VEGA project, the aim is to develop novel transfer learning methods to 3D geometric data. The main challenge is to create methods that can use information learned for a task in other contexts. In order for them to perform an action such as recognising a specific shape, neural networks - the basic systems in machine learning - must normally be trained on a very large quantity of 3D models. The transfer learning method consists of using a neural network that has already been "pre-trained" on a generic database and then fine-tuning it to adapt to novel problems and unseen shapes. This idea has already enabled the tremendous recent progress in image and text analysis and generation.
However, the machine learning methods that exist for 2D images are not directly transposable to 3D shapes and, when they are, it is often done in an "ad hoc" way, without researchers knowing exactly in which cases they can be used. One limitation is the small size of 3D databases to pre-train models. But there are also challenges related to the specificity of these data. In addition to having an extra dimension, 3D models rely much more on the notion of geometric structure than 2D images. They require a different mathematical formalism. The best way to mathematically represent 3D data on a computer, and to create algorithmic architectures capable of processing them, remains an open question. "In my research, I use a lot of tools from differential or Riemannian geometry for example. This mathematical modelling and analysis aspect is a great source of satisfaction in my work," emphasises Maks Ovsjanikov.