Hi! Paris: AI meets healthcare
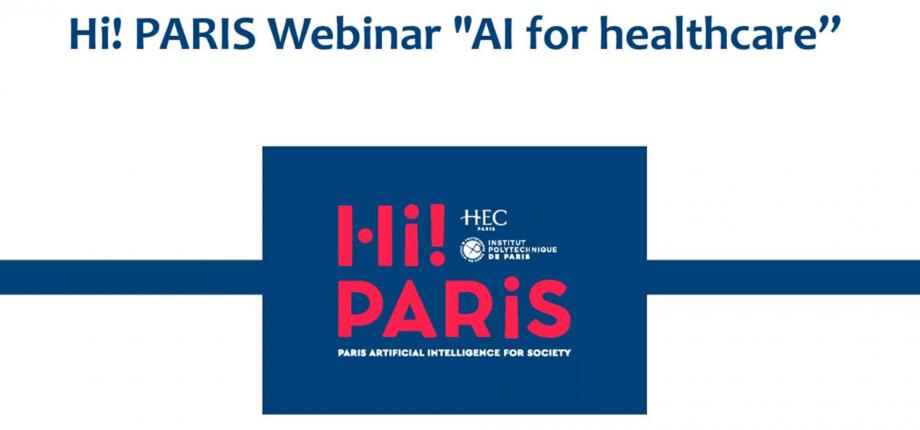
The global ambition of Hi! Paris interdisciplinary center is to ensure AI and data empower business and society. It takes advantage of cross-fertilization between fundamental sciences, technology, management and social sciences. In that context, AI research applications in healthcare are promising. Four scientists presented their current work during a research webinar hosted online on Friday, April 9th.
Disease diagnosis and monitoring
Catalin Fetita, Professor at Télécom SudParis and head of the ARMEDIA team in SAMOVAR Lab*, presented the applications of AI models in the quantification of lung lesions in fibrosing idiopathic interstitial pneumonia (FIIP). FIIP is a type of pneumonia that requires regular follow-up with CT-scans to assess lesions progression. The goal of this research is to develop an automated system for such a follow-up. Deep learning and more specifically convolutional neural networks were trained thanks to a database annotated by radiologists. Different architectures have been compared and promising results have been obtained in near real time, even though theoretical challenges remain regarding the model accuracy and stability for a potential translation to clinical routine. Moreover, this research could now extend to Covid-19 since it is suspected to evolve to FIIP in 10% to 30% of the severe cases.
Pharmacokinetic modeling
Marc Lavielle, Professor at Ecole Polytechnique, senior researcher at INRIA and Center for Applied Mathematics (CMAP*) focuses on a new algorithm for automatic model building in pharmacology. When a patient takes a drug, pharmacokinetic modeling aims at knowing how it is absorbed, distributed and eliminated by the body. For a single person, building such a mathematical model is rather easy and only depends on a few individual parameters such as the absorption rate. But in practice, pharmacology studies populations comprising many patients, whose parameters show variability. Mixed effect models are widely used for this approach but finding the right one is difficult. Marc Lavielle developed an algorithm to automatically build such a model, much faster than standard methods. It is already implemented in Monolix, a plateform used for pharmacokinetic modeling. The challenge now lies in its use for more complex models, with even more parameters, such as pharmacogenomics.
Assistive robotics
Adriana Tapus, who is part of the Autonomous systems and robotics team in Computer science and system engineering department (U2IS*), presented her work on assistive robotics. Human-centered robots capable of helping vulnerable people address their own needs are a viable complementary tool for healthcare professionals. However, robots require highly individualized models to guide their behavior. The research presented by Adriana Tapus covers different angles of this problem, such as user’s activity recognition, where the robot must identify in real time what the human is doing. AI long term convolutional networks have been developed to perform this task with a high successful recognition rate. Furthermore, researchers study how the user’s trust in the robot evolve, depending on how well the robot performs. Among future challenges, Adriana Tapus and her team are trying to adjust their AI model in order to continuously learn during the human-robot interaction.
AI and electronic health records
In the last presentation, Gaël Varoquaux, senior researcher at INRIA, discussed how AI could help extract useful information from electronic health records. Health research is typically performed on carefully curated data from closely-followed cohorts in clinical studies. This is a hard and slow process. What if we could extract complementary information from a more mundane source of data, such as hospitals bookkeeping, doctor’s prescriptions and observations? The challenge is that these data are « dirty », with lots of missing values and non-normalized information (the convention used may vary from one doctor to another). Furthermore, contrary to clinical trials, there is no control experiment and one cannot draw direct conclusions on treatment efficacy. Gaël Varoquaux showed how AI and machine learning come into play by providing powerful statistical methods to extract and analyse these “dirty” data. In particular, how machine learning can deal with missing values, typos and morphological variants.
Following the presentation, researchers were joined by Théophile Mohr Durdez, CEO at Volta Medical, Benjamin Farcy, data scientist at Capgemini and Harry Sokol, gastroenterologist at Saint-Antoine hospital in Paris for a panel discussion about the various challenges of AI in healthcare. They especially shared their views on how to bridge the gap between research projects, reality on the ground and practitioner’s needs.
*SAMOVAR: a research lab Télécom SudParis - Institut Polytechnique de Paris
CMAP: a joint research unit CNRS, École Polytechnique - Institut Polytechnique de Paris
U2IS: a research lab ENSTA Paris - Institut Polytechnique de Paris