ERC SINGER 101054787 ADG - Stochastic dynamics of sINgle cells : Growth, Emergence and Resistance
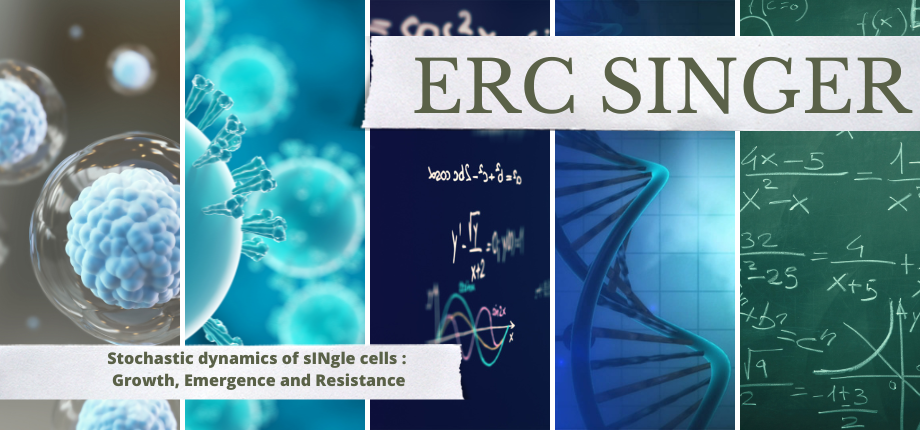
Thématiques
- Mathématiques appliquées
- Processus stochastiques
- Evolution bactérienne
- Modélisation en hématologie
- Biologie
- Recherche
- Médical
A propos
Le projet que porte l’ERC SINGER vise à développer de nouveaux modèles stochastiques et déterministes pour des applications biologiques et médicales. Il s’agit d’un défi sociétal interdisciplinaire qui va chercher à développer des scénarios d’évolution des résistances ainsi que de meilleures stratégies pour les antibiotiques ou les chimiothérapies qui y sont liés.
L’objectif principal consiste à étudier de nouveaux problèmes mathématiques à plusieurs échelles, en nous inspirant des défis biologiques posés par les organismes unicellulaires. En collaborant étroitement avec des experts en biologie, nous cherchons à comprendre et à modéliser la complexité des populations évolutives, tout en contribuant au développement de nouvelles théories mathématiques pour relever ces défis passionnants
Le projet
Le projet se concentre sur la biologie éco-évolutive des populations asexuées de bactéries ou de cellules, en explorant comment la survie et la reproduction dépendent des traits phénotypiques ou génétiques. L'évolution de ces traits résulte de divers mécanismes, et le défi majeur consiste à prédire les scénarios d'évolution en prenant en compte ces mécanismes. Le projet tire parti des mesures récentes de cellules individuelles, où de petites populations et une grande variabilité sont observées, avec un impact significatif de l'environnement.
L'objectif principal est de développer des modèles stochastiques pour contrôler ces fluctuations et prédire leur comportement en réponse aux changements environnementaux.
L'approche mathématique adoptée englobe une classe de modèles et caractéristiques, visant à des applications médicales et biologiques pour les populations évoluant, se reproduisant, croissant et émergeant. Une collaboration étroite avec des biologistes permettra de résoudre des problèmes biologiques en posant de nouvelles questions mathématiques. Le projet s'inscrit dans une tradition de développement de nouveaux concepts mathématiques à partir de découvertes biologiques, en utilisant des modèles de population structurés et de croissance-fragmentation.
La méthodologie implique des processus stochastiques structurés à valeur de mesure générant une diversité de comportements macroscopiques. Les principaux outils comprennent les théorèmes des limites, les équations différentielles stochastiques, les processus de ramification avec interactions et les équations différentielles partielles non linéaires.
Le projet représente un défi mathématique en cherchant à réconcilier les approches stochastiques et Hamilton-Jacobi dans la modélisation éco-évolutive, en explorant de nouvelles échelles de temps et de taille, et en contrôlant les événements d'extinction. Il aborde également des problèmes médicaux, notamment la compréhension des mutations leucémiques et des temps de réponse dans des systèmes multi-échelles, avec des implications potentielles pour les traitements médicaux.
Les thèmes de recherche clés :
la transition des processus stochastiques aux équations de Hamilton-Jacobi,
la modélisation des mutations leucémiques et des temps de réponse dans des systèmes multi-échelles,
la recherche de lignées et de chemins inversés dans des situations non linéaires,
la modélisation de la croissance et de la fragmentation des bactéries multitype en lien avec la réponse aux antibiotiques.
En résumé, ce projet s'appuie sur des modèles mathématiques pour explorer les mécanismes d'évolution des populations biologiques à différentes échelles, en mettant l'accent sur les défis mathématiques et les applications potentielles en biologie et en médecine.
Les 2 axes du projet
- Axe 1 : Développement d’un cadre mathématique pour suivre de très petites sous-populations dans des approximations de grandes populations afin d'expliquer et de quantifier des comportements surprenants à long terme dans la dynamique évolutive des populations, comme l'émergence de "populations éteintes"
- Axe 2 : Modélisation et compréhension mathématique des scénarios d'évolution des résistances en collaboration étroite avec les biologistes afin de proposer de nouvelles stratégies tenant compte de ces scénarios.
Le projet est supporté par :
“Funded by the European Union (ERC, SINGER, 101054787). Views and opinions expressed are however those of the author(s) only and do not necessarily reflect those of the European Union or the European Research Council Executive Agency. Neither the European Union nor the granting authority can be held responsible for them.”
Lien vers structures connexes
- Centre de Mathématiques Appliquées de l'Ecole Polytechnique
- Equipe de recherche PEIPS
Membres Permanents
Project Leader
- Sylvie Méléard, Professeur, Ecole polytechnique, France.
Mathématiciens
- Vincent Bansaye, Professeur, Ecole polytechnique, France.
- Nicolas Champagnat, DR, INRIA Nancy - Grand est, France.
- Marie Doumic, DR, INRIA Paris, France.
- Sepideh Mirrahimi, DR, CNRS Montpellier, France.
- Chi Viet Tran, Professeur, Université Gustave Eiffel, France.
- Anita Winter, Professeur, Universität Duisburg - Essen, Allemagne.
Biologistes et Médecins
- Sylvain Billiard, Professeur, Université de Lille, France.
- Meriem El Karoui, Professeur, Université d'Edimbourg, Ecosse.
- Stéphane Giraudier, Professeur, Hôpital Saint - Louis, France.
- Raphaël Itzykson, Professeur, Hôpital Saint - Louis, France.
- Evelyne Lauret, CR, CNRS, INSERM, Institut Cochin, France.
Membres temporaires
- Clément Foucart (01/09/2023 - 31/08/2025) chercheur associé
- Pierre Gabriel (01/02/2023 – 30/07/2023) délégation
- Sarah Kaakai (01/09/2023 - 31/08/2024) délégation
- Jaime San Martin (3/05/2023-05/07/2023) Professeur invité
Post-doctorant
- Laetitia Colombani (01/09/2023 - 24/08/2024), Ecole polytechnique, France.
- Manuel Esser (02/05/2025 - 30/09/2027), Ecole polytechnique, France.
Doctorants
- Anouar Jeddi (01/09/2024 - 31/08/2026), Ecole polytechnique, France.
- Alexandre Perrin (01/09/2024 - 31/08/2026), Ecole polytechnique, France.
- Deangeli Bravo Mateo (01/10/2024-01/10/2027), Ecole polytechnique, France.
Evènements à destination du public
- Séminaire
- Ecole d’été
Evènements internes
Groupe de travail
Séminaire au Château de Mée
Project Seminar and Workshop
Participants: Vincent Bansaye, Clément Foucart, Sylvie Méléard, Marie Doumic, Meriem El Karoui, Raphaël Itzykson, Carine Legrand, Michael Réra, Sarah Kaakai, Viet Chi Tran, Nicolas Champagnat, Sylvain Billiard, Anabely Gutierrez, Ana Fernandez Baranda, Luce Breuil, Alexandre Perrin, Mateo Deangeli Bravo.
Publications du projet
[1] N. Champagnat, S. Méléard, S. Mirrahimi, C.V. Tran. "Filling the gap between individual-based evolutionary models and Hamilton-Jacobi equations". J. de l'Ecole polytechnique- Mathematiques, (2023) Tome 10 p. 1247-1275.
[2] I. Madrid Canales (2023) "Exponential ergodicity of a degenerate age-size piecewise deterministic process." Acta Applicandae Mathematicae . 187:5.
[3] B.Henry, S. Méléard, C.V. Tran. "Time reversal of spinal processes for linear and non-linear branching processes near stationarity". Electron. J. Probab. 28: 1-27, 2023.
[4] N. Champagnat, D. Villemonais. "General criteria for the study of quasi-stationarity." Electron. J. Probab. 28 1 - 84, 2023.
à definir
Sylvie Méléard :
• Conférencière invitée à l'ICM 2022 : Londres 2022.
• Conférence Medaillon IMS : Conférence de probabilité appliquée INFORMS, Nancy 2023.
• Conférencière plénière. Panorama des mathématiques, Centre Hausdorff, Bonn 2023.
Membres Permanents
Project Leader
- Sylvie Méléard, Professeur, Ecole polytechnique, France.
Mathématiciens
- Vincent Bansaye, Professeur, Ecole polytechnique, France.
- Nicolas Champagnat, DR, INRIA Nancy - Grand est, France.
- Marie Doumic, DR, INRIA Paris, France.
- Sepideh Mirrahimi, DR, CNRS Montpellier, France.
- Chi Viet Tran, Professeur, Université Gustave Eiffel, France.
- Anita Winter, Professeur, Universität Duisburg - Essen, Allemagne.
Biologistes et Médecins
- Sylvain Billiard, Professeur, Université de Lille, France.
- Meriem El Karoui, Professeur, Université d'Edimbourg, Ecosse.
- Stéphane Giraudier, Professeur, Hôpital Saint - Louis, France.
- Raphaël Itzykson, Professeur, Hôpital Saint - Louis, France.
- Evelyne Lauret, CR, CNRS, INSERM, Institut Cochin, France.
Membres temporaires
- Clément Foucart (01/09/2023 - 31/08/2025) chercheur associé
- Pierre Gabriel (01/02/2023 – 30/07/2023) délégation
- Sarah Kaakai (01/09/2023 - 31/08/2024) délégation
- Jaime San Martin (3/05/2023-05/07/2023) Professeur invité
Post-doctorant
- Laetitia Colombani (01/09/2023 - 24/08/2024), Ecole polytechnique, France.
- Manuel Esser (02/05/2025 - 30/09/2027), Ecole polytechnique, France.
Doctorants
- Anouar Jeddi (01/09/2024 - 31/08/2026), Ecole polytechnique, France.
- Alexandre Perrin (01/09/2024 - 31/08/2026), Ecole polytechnique, France.
- Deangeli Bravo Mateo (01/10/2024-01/10/2027), Ecole polytechnique, France.
Evènements à destination du public
- Séminaire
- Ecole d’été
Evènements internes
Groupe de travail
Séminaire au Château de Mée
Project Seminar and Workshop
Participants: Vincent Bansaye, Clément Foucart, Sylvie Méléard, Marie Doumic, Meriem El Karoui, Raphaël Itzykson, Carine Legrand, Michael Réra, Sarah Kaakai, Viet Chi Tran, Nicolas Champagnat, Sylvain Billiard, Anabely Gutierrez, Ana Fernandez Baranda, Luce Breuil, Alexandre Perrin, Mateo Deangeli Bravo.
Publications du projet
[1] N. Champagnat, S. Méléard, S. Mirrahimi, C.V. Tran. "Filling the gap between individual-based evolutionary models and Hamilton-Jacobi equations". J. de l'Ecole polytechnique- Mathematiques, (2023) Tome 10 p. 1247-1275.
[2] I. Madrid Canales (2023) "Exponential ergodicity of a degenerate age-size piecewise deterministic process." Acta Applicandae Mathematicae . 187:5.
[3] B.Henry, S. Méléard, C.V. Tran. "Time reversal of spinal processes for linear and non-linear branching processes near stationarity". Electron. J. Probab. 28: 1-27, 2023.
[4] N. Champagnat, D. Villemonais. "General criteria for the study of quasi-stationarity." Electron. J. Probab. 28 1 - 84, 2023.
à definir
Sylvie Méléard :
• Conférencière invitée à l'ICM 2022 : Londres 2022.
• Conférence Medaillon IMS : Conférence de probabilité appliquée INFORMS, Nancy 2023.
• Conférencière plénière. Panorama des mathématiques, Centre Hausdorff, Bonn 2023.